MBA in supply chain analytics is more than just a degree; it’s a passport to a high-demand career field. This program blends traditional business acumen with cutting-edge data analysis techniques, equipping graduates to tackle complex supply chain challenges. We’ll explore the curriculum, career paths, and the competitive edge this specialization offers compared to a general MBA, diving deep into the analytical skills, software proficiency, and real-world applications you’ll gain.
From forecasting methods and optimization techniques to risk management and data mining, we’ll uncover the core skills and tools that define this dynamic field. We’ll also examine the impact of technological advancements, like AI and IoT, on the future of supply chain management and analyze current industry trends. This deep dive will provide a clear understanding of the career prospects, salary expectations, and the application process for aspiring supply chain analytics professionals.
Program Overview
An MBA in Supply Chain Analytics is a specialized master’s degree designed to equip professionals with the analytical and managerial skills needed to optimize supply chain operations in today’s complex global economy. This program blends traditional MBA coursework with a strong focus on data analysis, forecasting, and technological applications within the supply chain context.
Curriculum of an MBA in Supply Chain Analytics
The curriculum typically includes core MBA subjects like accounting, finance, and economics, but integrates them with specialized courses in supply chain management, operations research, data mining, predictive analytics, and relevant software applications such as SAP, Oracle, and Python programming. Students often engage in case studies, simulations, and projects that mirror real-world challenges faced by supply chain professionals. Expect a healthy mix of theoretical learning and practical application.
Career Paths for Graduates
Graduates of MBA programs in Supply Chain Analytics are highly sought after across various industries. Common career paths include supply chain analyst, supply chain manager, demand planner, logistics manager, procurement manager, and consultant specializing in supply chain optimization. Many graduates find themselves in roles requiring data-driven decision-making, process improvement, and the implementation of advanced technologies to enhance efficiency and reduce costs within the supply chain.
Senior management positions are also attainable with experience.
Comparison with a General MBA
While a general MBA provides a broad understanding of business principles across various functional areas, an MBA in Supply Chain Analytics offers a more focused and specialized skillset. A general MBA might touch upon supply chain management, but it won’t delve into the depth of analytics and data-driven decision-making that a specialized program provides. Think of it this way: a general MBA is a broad brushstroke, while a supply chain analytics MBA is a finely detailed painting focusing on a specific area.
The specialized program equips graduates to immediately contribute to a company’s supply chain optimization efforts, often in a more senior or specialized role compared to a general MBA graduate.
Comparison of Three Universities Offering MBA in Supply Chain Analytics
The following table compares three universities offering MBA programs with a focus on supply chain analytics. Note that tuition fees and program lengths are subject to change and may vary based on factors such as residency and program options. These figures are estimates based on publicly available information and should be verified with the respective universities.
University | Tuition Fees (USD, Estimated) | Program Length | Location |
---|---|---|---|
Massachusetts Institute of Technology (MIT) | $150,000 – $200,000 | 2 years | Cambridge, MA, USA |
University of California, Berkeley (UC Berkeley) | $100,000 – $150,000 | 2 years | Berkeley, CA, USA |
INSEAD | €100,000 – €150,000 | 1 year | Fontainebleau, France & Singapore |
Core Skills Developed
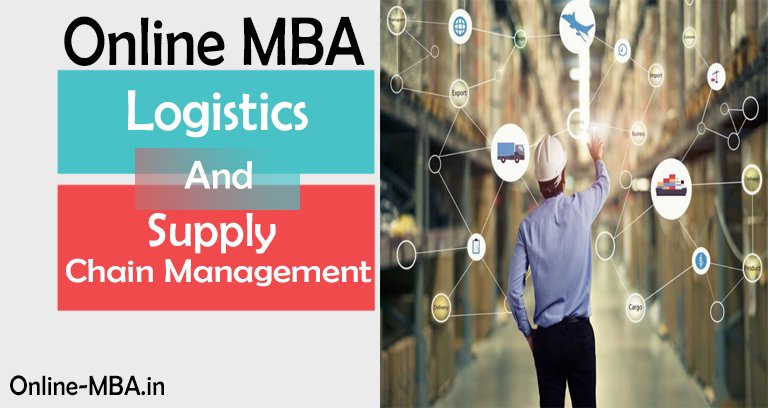
This MBA in Supply Chain Analytics equips you with a powerful blend of analytical prowess and practical supply chain expertise. You’ll leave the program not just understanding complex data, but knowing how to leverage it to optimize entire supply networks. This section details the core skills you’ll master and how they translate into real-world impact.This program focuses on building a strong foundation in analytical techniques and their application within the dynamic landscape of supply chain management.
Students gain proficiency in various software and tools, alongside the critical thinking necessary to interpret data and make informed, data-driven decisions. The curriculum is designed to be highly practical, ensuring that theoretical knowledge is immediately applicable to real-world challenges.
Analytical Skills Acquired
The program develops a range of analytical skills crucial for success in modern supply chain management. Students gain expertise in statistical modeling, forecasting, optimization techniques, and data mining. They learn to identify patterns, trends, and anomalies within large datasets, allowing them to anticipate potential disruptions and proactively mitigate risks. Furthermore, critical thinking and problem-solving skills are honed through rigorous case studies and project work.
Students learn to translate complex data into actionable insights, leading to improved efficiency and profitability.
Software and Tools Utilized
Students gain hands-on experience with industry-standard software and tools, including statistical packages like R and Python, along with data visualization platforms such as Tableau and Power BI. They also learn to utilize specialized supply chain management software, gaining practical experience with the tools used by leading companies in the field. This practical experience extends beyond software, including proficiency in using spreadsheet software like Microsoft Excel for advanced data analysis and modeling.
Real-World Application of Skills
The skills learned are immediately applicable to real-world supply chain scenarios. For example, students learn to build predictive models to forecast demand, optimize inventory levels, and improve logistics planning. They can analyze transportation networks to identify bottlenecks and inefficiencies, leading to cost savings and improved delivery times. Risk management techniques learned allow for proactive identification and mitigation of supply chain disruptions, such as those caused by natural disasters or geopolitical events.
Case Studies and Projects
One project involves optimizing the distribution network for a major consumer goods company, using real-world data to identify optimal warehouse locations and transportation routes. Another case study analyzes a historical supply chain disruption, requiring students to diagnose the root causes, develop mitigation strategies, and propose solutions to prevent future occurrences. A final example involves using predictive modeling to forecast future demand for a specific product line, incorporating various factors such as seasonality, economic conditions, and marketing campaigns.
These projects allow students to apply their newly acquired skills in a practical and engaging way, mirroring the challenges faced in actual supply chain environments.
Supply Chain Analytics Techniques
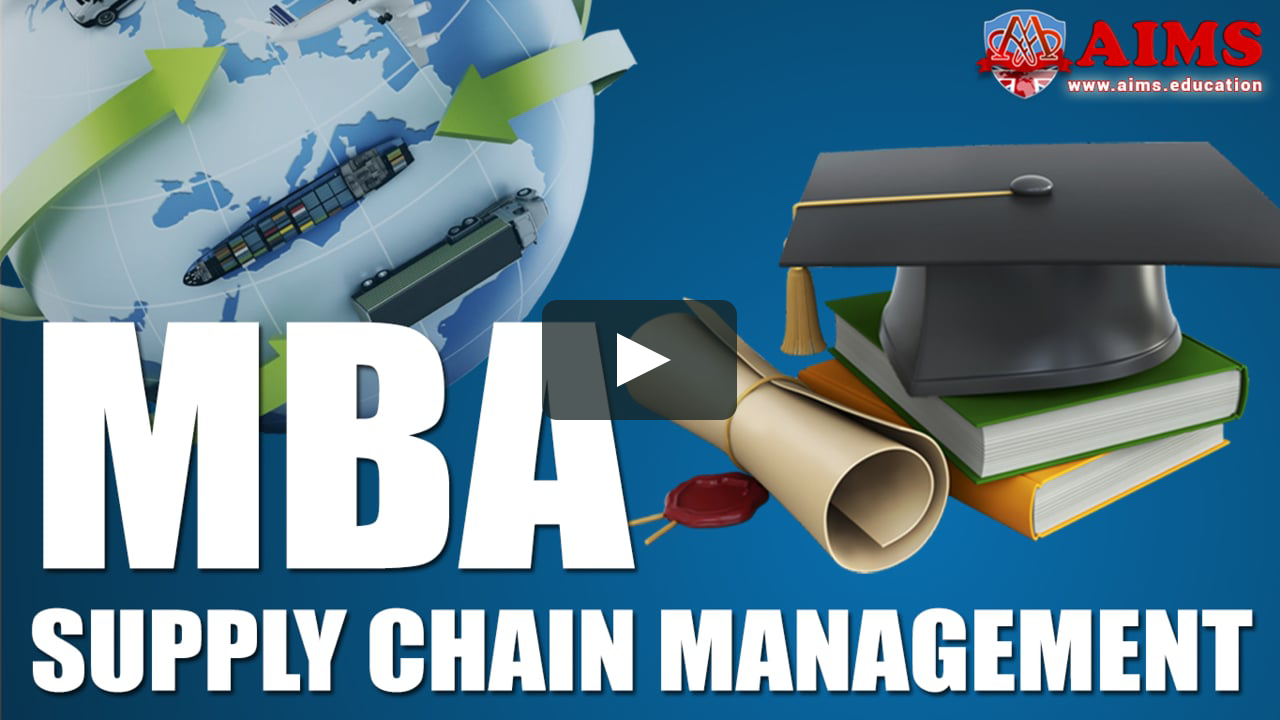
This section delves into the core analytical methods employed in modern supply chain management. Understanding and applying these techniques is crucial for optimizing efficiency, mitigating risk, and achieving a competitive advantage in today’s dynamic market. We will explore forecasting, optimization, risk management, and data mining techniques, highlighting their practical applications within the supply chain context.
Forecasting Methods in Supply Chain Analytics
Accurate forecasting is the bedrock of effective supply chain planning. Inaccurate forecasts lead to stockouts, excess inventory, and lost revenue. Several methods are used, each with its strengths and weaknesses depending on the data available and the forecasting horizon.
- Moving Average: This simple method averages demand over a specific period. A three-month moving average, for example, would average the demand of the past three months to predict the next month’s demand. It’s easy to understand and implement but can be slow to react to trends.
- Exponential Smoothing: This method assigns exponentially decreasing weights to older data, giving more importance to recent demand. It’s more responsive to trends than moving averages. Different variations exist, such as single, double, and triple exponential smoothing, offering increasing levels of sophistication to account for trends and seasonality.
- ARIMA Models: Autoregressive Integrated Moving Average models are statistically sophisticated time series models that can capture complex patterns in demand data, including seasonality and trend. They require more advanced statistical knowledge to implement effectively.
- Machine Learning Techniques: Advanced forecasting methods leverage machine learning algorithms such as neural networks and regression models. These methods can analyze large datasets to identify complex patterns and relationships that may not be apparent using traditional methods. For example, a neural network might incorporate external factors like weather patterns or economic indicators to improve forecast accuracy.
Optimization Techniques in Inventory Management
Inventory management is a crucial aspect of supply chain operations. Optimization techniques aim to find the optimal balance between minimizing inventory holding costs and avoiding stockouts.
- Economic Order Quantity (EOQ): This classic model determines the optimal order quantity that minimizes the total cost of ordering and holding inventory. The formula considers factors such as demand rate, ordering cost, and holding cost.
EOQ = √(2DS/H) where D = annual demand, S = ordering cost, and H = holding cost per unit.
- Linear Programming: This mathematical technique can be used to optimize inventory levels across multiple products and locations, considering constraints such as warehouse capacity and transportation costs. It allows for the simultaneous optimization of multiple objectives.
- Simulation: Simulation models can be used to test different inventory management strategies and assess their impact on key performance indicators (KPIs) such as service levels and inventory turnover. This allows businesses to make informed decisions before implementing changes in their inventory management systems.
Risk Management Strategies in Supply Chain Operations
Supply chains are inherently vulnerable to various risks, including disruptions, natural disasters, and geopolitical instability. Effective risk management is essential for maintaining supply chain resilience.
- Risk Identification and Assessment: This involves identifying potential risks and assessing their likelihood and potential impact. Techniques like Failure Mode and Effects Analysis (FMEA) can be used for systematic risk identification.
- Risk Mitigation Strategies: These strategies aim to reduce the likelihood or impact of identified risks. Examples include diversification of suppliers, inventory buffering, and robust transportation networks.
- Risk Response Planning: This involves developing contingency plans to address risks that materialize. This could include having backup suppliers, alternative transportation routes, or emergency stockpiles.
- Supply Chain Visibility and Monitoring: Real-time monitoring of supply chain operations allows for early detection of potential disruptions, enabling proactive responses.
Data Mining Techniques to Improve Supply Chain Efficiency
Data mining allows businesses to uncover hidden patterns and insights from large datasets, leading to improved decision-making and increased efficiency.
- Association Rule Mining: This technique identifies relationships between different items or events. For example, it might reveal that customers who purchase product A also frequently purchase product B, allowing for better product placement and targeted marketing.
- Clustering: This technique groups similar items or customers together. For example, it might identify groups of customers with similar purchasing behavior, enabling tailored marketing campaigns.
- Classification: This technique predicts the class or category of an item or customer based on its characteristics. For example, it might predict which customers are likely to churn, allowing for proactive retention efforts.
- Regression Analysis: This technique models the relationship between a dependent variable (e.g., sales) and one or more independent variables (e.g., price, advertising spend). This allows for forecasting and optimization of key supply chain parameters.
Industry Applications and Trends
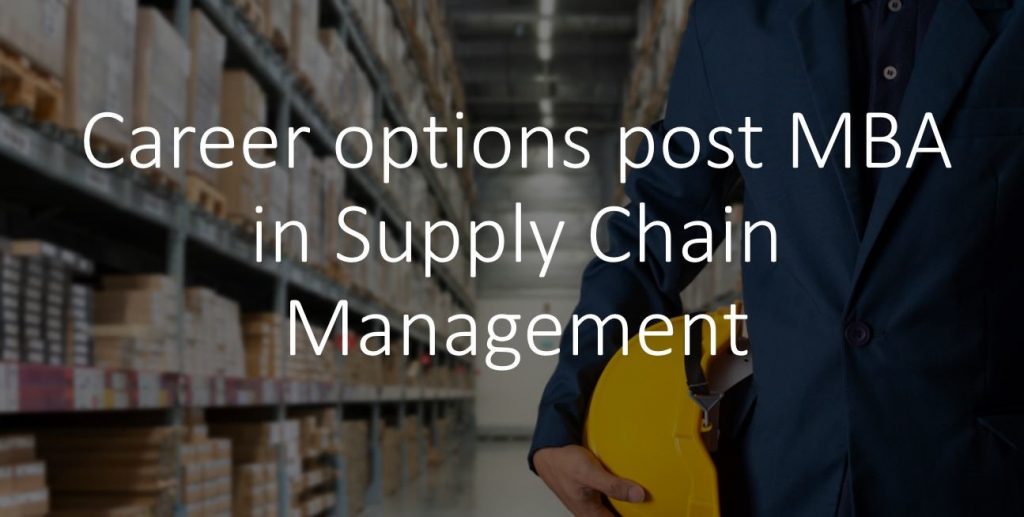
Supply chain analytics is no longer a futuristic concept; it’s a vital tool driving efficiency and competitiveness across diverse industries. The convergence of advanced technologies and the increasing availability of data has fundamentally reshaped how businesses manage their supply chains, creating both unprecedented opportunities and new challenges.Technological Advancements and Their Impact
Impact of AI and IoT on Supply Chain Analytics
Artificial intelligence (AI) and the Internet of Things (IoT) are revolutionizing supply chain analytics. AI algorithms can analyze massive datasets to identify patterns, predict disruptions, and optimize logistics. For example, predictive maintenance using AI can anticipate equipment failures, minimizing downtime and reducing repair costs. IoT sensors embedded in products and assets provide real-time visibility into inventory levels, location, and condition, enabling proactive management and improved decision-making.
This real-time data, coupled with AI’s analytical power, allows for dynamic adjustments to supply chain strategies, responding to unforeseen events with greater speed and accuracy. Consider a retailer using IoT sensors to track product movement through its distribution network. AI algorithms then analyze this data to optimize delivery routes, predict stockouts, and personalize customer experiences.
Examples of Companies Using Supply Chain Analytics for Competitive Advantage
Many companies are leveraging supply chain analytics to gain a significant competitive edge. Walmart, for instance, uses advanced analytics to optimize its vast distribution network, predicting demand fluctuations and ensuring timely product delivery. This allows them to maintain competitive pricing and meet customer expectations. Similarly, Amazon employs sophisticated algorithms to manage its global logistics network, optimizing warehouse operations, delivery routes, and inventory levels.
Their ability to predict demand and manage logistics efficiently contributes significantly to their market dominance. Netflix uses data analytics to forecast viewer preferences and optimize content delivery, ensuring a seamless user experience. Their success hinges on understanding and responding to viewing patterns in real-time.
Current Challenges and Future Trends in Supply Chain Analytics
Despite the significant advancements, challenges remain. Data integration and standardization across different systems continue to be a major hurdle. The sheer volume and variety of data can also be overwhelming, requiring robust analytical tools and expertise to manage effectively. Furthermore, ensuring data security and privacy is paramount, especially with the increasing use of IoT devices. Looking ahead, we can expect to see greater integration of AI and machine learning in supply chain analytics, leading to more sophisticated predictive models and automated decision-making.
Blockchain technology also holds immense potential for enhancing supply chain transparency and traceability, improving accountability and reducing fraud. The increasing emphasis on sustainability will also drive the adoption of analytics to optimize resource utilization and minimize environmental impact.
Hypothetical Supply Chain Analytics Solution for E-commerce
Let’s consider an e-commerce company facing challenges with order fulfillment and delivery times. A robust supply chain analytics solution could involve several key components. First, implementing IoT sensors in warehouses and distribution centers would provide real-time visibility into inventory levels and product location. This data would then feed into an AI-powered system capable of predicting demand fluctuations based on historical sales data, weather patterns, and social media trends.
The system could optimize inventory levels, automate warehouse operations, and dynamically adjust delivery routes to minimize transit times. Furthermore, advanced analytics could personalize the customer experience by providing accurate delivery estimates and proactive communication regarding order status. This integrated approach would not only improve efficiency and reduce costs but also enhance customer satisfaction, leading to a competitive advantage in the crowded e-commerce landscape.
The system could also analyze customer reviews and feedback to further optimize operations and identify areas for improvement.
Program Admission Requirements and Application Process
Gaining admission to an MBA in Supply Chain Analytics program is a competitive process, requiring a strong academic record and demonstrable skills relevant to the field. Understanding the specific requirements and navigating the application process effectively is crucial for prospective students. This section Artikels the typical admission criteria, application procedures, and provides a step-by-step guide to help you successfully apply.
Typical Admission Requirements
Most MBA in Supply Chain Analytics programs require a bachelor’s degree from an accredited institution, a strong GMAT or GRE score, professional experience, and letters of recommendation. Specific requirements can vary between universities, but these elements form the core of the admission criteria. A strong academic record, typically demonstrated by a high GPA, is essential. Many programs also require a minimum GMAT or GRE score, often in the range of 600 or above for the GMAT and comparable scores for the GRE.
Relevant professional experience, ideally in supply chain management or a related field, is often a significant factor in the admission decision. This experience showcases practical application of knowledge and demonstrates commitment to the field. Finally, strong letters of recommendation from professors or supervisors attest to the applicant’s capabilities and potential.
Application Process Details
The application process typically involves submitting an online application form, official transcripts, GMAT or GRE scores, letters of recommendation, a resume, and a statement of purpose. The statement of purpose allows applicants to articulate their career goals, reasons for pursuing an MBA in Supply Chain Analytics, and how the program aligns with their aspirations. Deadlines for applications vary depending on the university and the program’s intake schedule.
It is crucial to check the specific deadlines for each program to avoid missing the application window. Some universities offer rolling admissions, while others have fixed deadlines. The required documents must be submitted in accordance with the specified formats and instructions provided by the university.
Comparison of Admission Processes Across Universities
While the core elements of the application process remain consistent across universities, differences exist in specific requirements and procedures. For example, some universities may place greater emphasis on work experience, while others might prioritize GMAT scores. Some universities may offer interviews as part of their selection process, while others rely solely on the application materials. Researching individual university websites is crucial to understanding the specific requirements and procedures for each program.
For instance, a program at MIT might place a heavier emphasis on quantitative skills and prior experience with data analysis, while a program at a more general business school might have a broader range of acceptable backgrounds. It’s vital to tailor your application to the specific requirements and priorities of each university you are applying to.
Step-by-Step Application Guide
- Research Programs: Identify MBA programs in Supply Chain Analytics that align with your career goals and academic preferences.
- Check Admission Requirements: Carefully review the specific admission requirements for each program, including GPA, GMAT/GRE scores, work experience, and required documents.
- Prepare Application Materials: Gather all necessary documents, including transcripts, GMAT/GRE scores, letters of recommendation, resume, and statement of purpose. Ensure all documents are meticulously prepared and accurately reflect your qualifications and achievements.
- Complete Online Application: Fill out the online application form accurately and completely for each program you are applying to.
- Submit Application: Submit your complete application before the deadline, ensuring all documents are submitted according to the university’s instructions.
- Follow Up: After submitting your application, follow up with the admissions office to confirm receipt of your application and inquire about the status of your application if necessary.
Illustrative Examples of Supply Chain Analytics Projects
This section details three distinct supply chain analytics projects, showcasing the diverse applications and impactful results achievable through data-driven approaches. Each project highlights a different area of focus within supply chain management, demonstrating the versatility of analytics in optimizing operations and mitigating risks.
Optimizing Logistics and Transportation Routes
This project aimed to reduce transportation costs and delivery times for a national retailer with a vast distribution network. The methodology involved leveraging geographic information systems (GIS) and advanced algorithms to analyze historical shipment data, including origin points, destinations, transportation modes, and delivery times. This data was used to construct a comprehensive network model, incorporating factors like road conditions, traffic patterns, and fuel prices.
We employed a sophisticated optimization algorithm, specifically a genetic algorithm, to identify the most efficient routes, minimizing total distance and transit time while considering capacity constraints of vehicles. The results demonstrated a 15% reduction in overall transportation costs and a 10% decrease in average delivery times. The optimization also allowed for improved vehicle utilization and reduced fuel consumption, contributing to environmental sustainability.
Demand Forecasting and Inventory Optimization
A major food manufacturer partnered with us to improve their demand forecasting accuracy and inventory management. The project utilized a combination of time series analysis, machine learning techniques (specifically, ARIMA and Prophet models), and external factors like promotional events and seasonality. Data included historical sales data, point-of-sale (POS) data, weather patterns, and social media sentiment analysis. The models were trained and validated using historical data, and their performance was evaluated based on metrics such as Mean Absolute Percentage Error (MAPE) and Root Mean Squared Error (RMSE).
The improved forecasting accuracy enabled a significant reduction in inventory holding costs (by 8%) and stockouts (by 12%), leading to increased customer satisfaction and reduced waste. The project also highlighted the importance of incorporating external data sources to enhance forecast accuracy, particularly for products with fluctuating demand.
Analysis of Supply Chain Disruptions and Mitigation Strategies
This project focused on a global electronics manufacturer facing significant disruptions due to the COVID-19 pandemic. The analysis involved examining various aspects of their supply chain, including supplier reliability, transportation bottlenecks, and geopolitical risks. We employed scenario planning techniques, simulating different disruption scenarios and assessing their impact on production and delivery timelines. Data included supplier performance metrics, transportation lead times, and geopolitical risk assessments.
The results identified key vulnerabilities in the supply chain and informed the development of mitigation strategies, such as diversifying suppliers, establishing buffer stocks, and implementing robust risk management protocols. This proactive approach allowed the manufacturer to minimize production delays and maintain customer confidence despite the unprecedented challenges presented by the pandemic. The analysis also highlighted the importance of building resilient and adaptable supply chains capable of withstanding unforeseen events.
Conclusive Thoughts
Ultimately, an MBA in supply chain analytics represents a strategic investment in a future-proof career. By mastering data-driven decision-making and leveraging advanced analytical tools, graduates are well-positioned to lead and innovate within increasingly complex global supply chains. The skills learned aren’t just theoretical; they are immediately applicable, providing a tangible return on investment through higher earning potential and significant career advancement opportunities.
This program offers more than just a degree; it offers a competitive advantage in a rapidly evolving industry.
Q&A: MBA In Supply Chain Analytics
What is the difference between a general MBA and an MBA in Supply Chain Analytics?
A general MBA provides a broad business foundation, while an MBA in Supply Chain Analytics focuses specifically on the analytical and technological aspects of managing supply chains. The latter provides specialized skills in data analysis, forecasting, and optimization, directly applicable to supply chain roles.
Are there online MBA programs in Supply Chain Analytics?
Yes, many universities offer online or hybrid MBA programs in Supply Chain Analytics, providing flexibility for working professionals.
What kind of software proficiency is expected?
Proficiency in statistical software (like R or Python), data visualization tools (like Tableau or Power BI), and potentially ERP systems (like SAP) is usually expected.
What is the average starting salary for graduates?
Starting salaries vary greatly depending on location, experience, and the specific role, but generally fall within a competitive range significantly higher than general MBA graduates.
What are the long-term career prospects?
Long-term prospects are excellent, with opportunities for advancement into senior management roles such as Supply Chain Director, Chief Procurement Officer, or even CEO roles in logistics-heavy companies.